June 19, 2023
Artificial intelligence promises tremendous benefits — from personalized recommendations to predictive analytics and automated decision-making. However, many companies rush into AI initiatives without considering the data infrastructure needed to support reliable and impactful AI models over the long term.
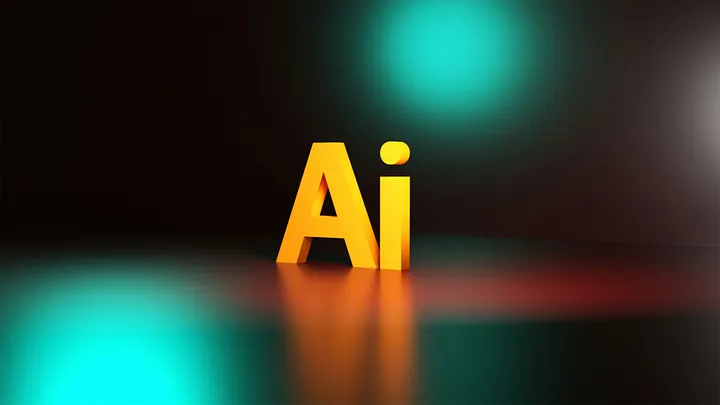
Curabitur Pellentesque Odio Magna
Donec luctus orci elit, vel mollis nisl cursus sit amet. Nam ut enim bibendum, placerat nulla ac, vestibulum urna. Praesent enim erat, consectetur vitae tempus sed, dapibus id nunc. Maecenas in purus cursus, efficitur massa nec, ullamcorper arcu. Integer justo nib suscipit ut tincidunt ut, blandit id quam. Maecenas et cursus metus. Aenean luctus consequat sapien, eget accumsan justo.
As a result, most AI projects fail to move beyond proofs-of-concept and pilots, leaving their transformative potential untapped. A recent Gartner survey found that by 2025, 85% of big data and AI projects will deliver erroneous outcomes due to bias in data, algorithms, or both.
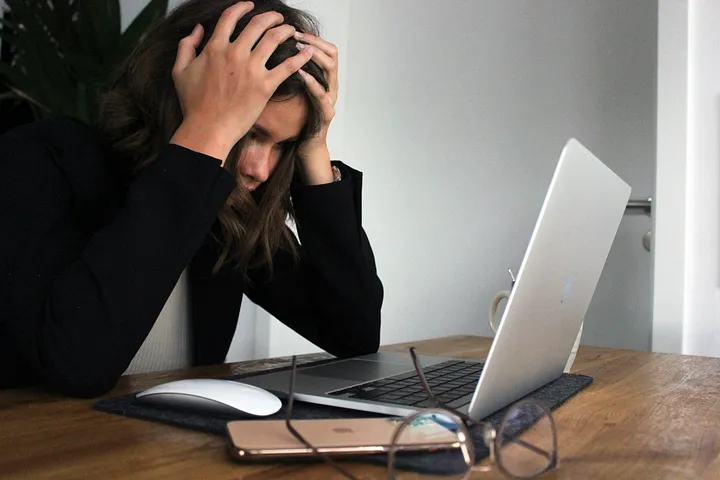
The core issue is that data serves as the fuel for any AI system. Without high-quality, reliable, and properly governed data pipelines, AI models deliver low-value insights that degrade in accuracy over time or become unusable.
That’s why forward-thinking companies like ITM are advocating a “data-first” approach to AI — with a sharp focus on building reusable data infrastructure through sound data engineering principles and architecture before diving into advanced analytics and machine learning.
The Power of Iterative Data Foundations
ITM’s data engineers have years of hands-on experience building tailored data platforms and pipelines for companies across retail, manufacturing, healthcare, and more industries. Their approach is rooted in starting small, failing fast, and scaling impact — enabling clients to maximize returns while minimizing risks.
Here are a few examples of how ITM helped clients build iteratively on data:
Retail Data Consolidation — A Major Home Goods Retailer
A major home goods retailer wanted to optimize its supply chain and inventory management by applying predictive analytics. However, its data was fragmented across multiple regional systems and warehouses with no cohesive architecture.
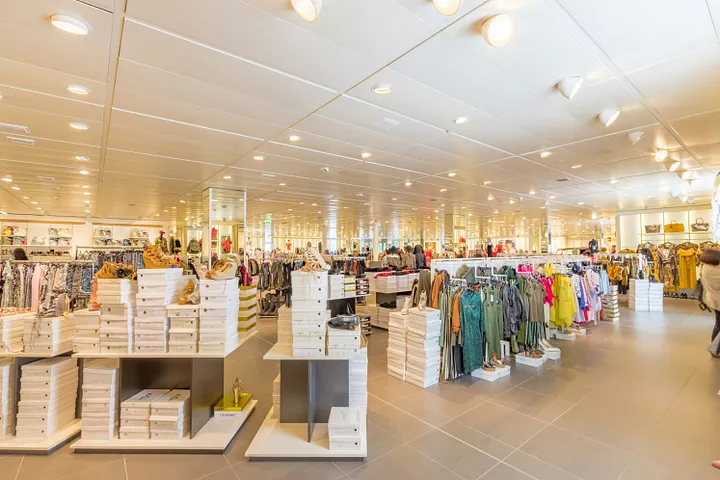
Rather than an expensive rip-and-replace integration, ITM designed a scalable approach to gradually consolidate and restructure the retailer’s data assets into a reusable central data lake. Standard interfaces enabled analysts to easily construct datasets tailored to different analytics use cases.
With higher-quality aggregated data, the retailer could start experimenting with demand forecasting algorithms at a few locations before expanding the capabilities chainwide. This minimized costs and risks while accelerating their AI journey.
Smart Manufacturing Use Cases — An Industrial Equipment Manufacturer
An industrial manufacturer wanted to leverage IoT sensor data from across its 30+ plants to reduce unplanned downtime and optimize quality testing. However, it lacked the modern data infrastructure to harness insights from vast amounts of streaming sensor data.
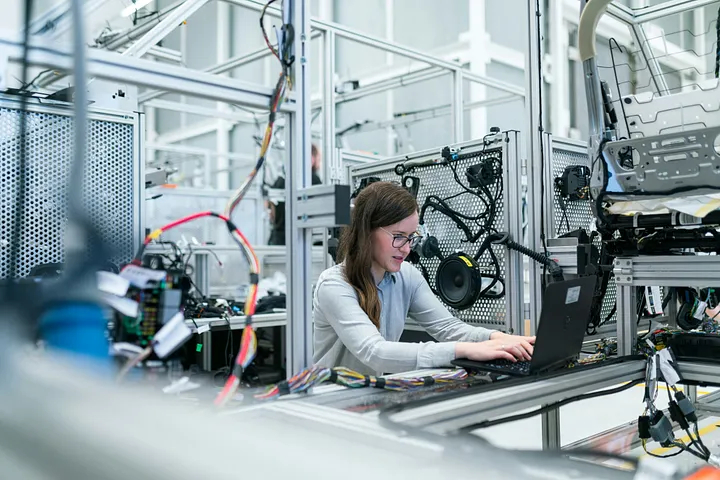
ITM engineered a cloud-based data platform to ingest and process high-velocity sensor streams using scalable architecture like Kafka, Spark and object storage. The derived datasets fueled ML models for early failure prediction and helped justify sensor investments to leadership.
By taking an iterative, use case-driven approach, the manufacturer could expand its platform’s capabilities over time to manage other data sources like supply chain systems and equipment logs for a true 360-degree production view.
Population Health Management — A Regional Hospital Network
A hospital network wanted to shift towards value-based care models, which reimburse providers based on health outcomes vs. services performed. This required analyzing clinical, claims, and patient data to identify high-risk patients and customize interventions.
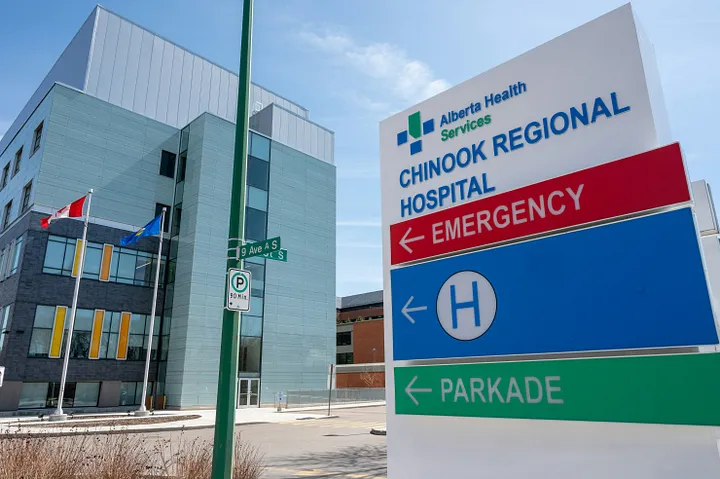
However, integrating the network’s fragmented health records, insurance claims, and external socioeconomic data posed regulatory and technical challenges. ITM implemented a HIPAA-compliant data lake to ingest, process, and govern access to these sensitive datasets at scale.
With clean, integrated data on social determinants and risk factors, analysts could better understand factors driving adverse outcomes. As a result, the network improved its risk-scoring model by 45% in the first year — enabling more targeted outreach and preventative care.
With clean, integrated data on social determinants and risk factors, analysts could better understand factors driving adverse outcomes. As a result, the network improved its risk-scoring model by 45% in the first year — enabling more targeted outreach and preventative care.
The Power of a Data-First Foundation
These examples highlight the transformative impact of investing in reusable data infrastructure before diving into AI. With strong data foundations, companies can rapidly iterate to produce high-impact analytics and models while controlling costs and risks.
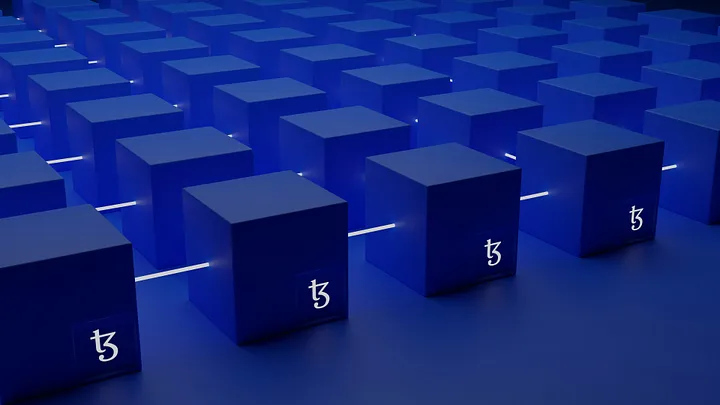
Unfortunately, many firms still believe that purchasing an off-the-shelf AI product or platform will somehow magically grant them cutting-edge capabilities overnight. As a result, they end up locked into costly tools that deliver little tangible value.
That’s why leading data-centric firms like ITM advocate getting back to basics — taking the time to understand business challenges, map out available data assets, architect integrated pipelines, and establish governance. This deliberate approach sets companies up for AI success over the long haul.
By skillfully navigating clients through the complexities of modern data architecture, ITM continues to disrupt traditional thinking around AI adoption. Their data foundations unlock innovation that evolves alongside business needs — transforming AI from a buzzword into a competitive advantage.